Free energy predictions are well established as a robust and accurate method of triaging potential drug candidates and guiding optimisation. Now the synergistic application of AI-based generative chemistry to free energy methods promises to streamline and industrialise molecule discovery and optimisation.
Drug discovery has always been an extremely difficult field. The list of criteria that a successful drug must fulfil is often very long, and many of them are rooted in the complex interplay of chemistry with biology. As a result, the optimisation of an initial hit molecule into a clinical candidate has always been at least in part a numbers game: you keep making changes until you get lucky.
The intuition and experience of a good medicinal chemist can certainly increase the chances of success, as can the predictions from computational chemistry models, but neither of these are close to infallible. However, the adoption of free energy perturbation calculations (FEP) over the last decade or so has been game changing. One of the key properties needed in a drug molecule, the ability to bind strongly to the target protein, is now amenable to calculation with a good degree of predictive accuracy.
Every large pharma organisation has invested significant resources in running FEP calculations because they can efficiently answer the primary question in drug development: how well is this molecule going to bind to my protein target?
Free energy methods involve simulating the behaviour of a molecule both bound to a protein and solvated in water which, together with a variety of thermodynamic tricks, allow the binding constant to be either calculated directly or indirectly by comparison to a related molecule whose binding is known. These methods have a long pedigree: the theoretical foundations were put in place nearly three quarters of a century ago.1
However, there were always two major difficulties in making use of the method. The first is our modelling of chemistry: we need to be able to compute the energy of organic molecules with a high degree of accuracy, and to do so extremely quickly. Quantum mechanics is accurate but not quick, so we rely on approximate classical methods, and these require extensive parameterisation to get sufficiently accurate answers.
The second is sampling: for accurate results, you need to explore all of the different configurations that the system can take up. For a small molecule in vacuum that is not too challenging, but adding water and including a large binding partner such as a protein makes sampling much more difficult. It is only relatively recently, with the advent of fast and relatively affordable GPUs, that free energy methods have moved from specialist calculations that required significant time on a supercomputer to everyday jobs run on a handful of computers (or even a single workstation).
The hard questions around druglikeness, metabolism, pharmacokinetics, toxicity, crystal polymorphs and so forth are still only loosely amenable to computational prediction, if at all.
This expansion of the use of free energy methods is enabled by widening their domain of applicability. The original methods have been expanded to solve technical problems around the calculation of binding affinities on charged molecules, peptides, and macrocycles, as well as handling complex cases where multiple tautomers and/or protomers can contribute to binding.2 The range of acceptable targets has also expanded to include membrane-bound proteins as well as DNA/RNA binders and disordered proteins.3 Exciting work is taking place on alternative ways of performing free energy calculations, such as alchemical transfer methods,4 multistate free energy methods,5 nonequilibrium methods6 and more. Access to these methods is also being simplified with the release of well-tested open-source frameworks for these calculations, such as SIRE7 and the Open Free Energy consortium.
In the last few years, the democratisation of free energy calculations has led to an explosion in their use in drug discovery. Every large pharma organisation has invested significant resources in running FEP calculations because they can efficiently answer the primary question in drug development: how well is this molecule going to bind to my protein target?
That is not to say that small molecule drug discovery can now be run entirely computationally: the hard questions around druglikeness, metabolism, pharmacokinetics, toxicity, crystal polymorphs and so forth are still only loosely amenable to computational prediction, if at all. However, when optimising for these properties being able to rule out, with a high degree of accuracy, which modifications to the lead molecule will harm the primary binding endpoint and which are acceptable (and being able to predict selectivity against related antitargets ahead of time) can lead to startling efficiency gains in the overall hit-to-lead and lead optimisation process. This can introduce significant savings in the very expensive and time-consuming drug discovery process.
Advances in available computing power have also contributed to the increasing industrialisation of the drug discovery process. Beginning in the late 1990s the focus started to shift from the careful, handcrafted synthesis of individually designed molecules towards bulk synthesis in combinatorial libraries. However, this was always limited by the inability to focus libraries effectively on the useful portion of chemistry space. Even with the inherent efficiencies of combinatorial synthesis, the cost (of both money and time) of synthesising and assaying large numbers of molecules that failed to reach the desired efficacy threshold was significant.
An important factor in facilitating the widespread use of free energy methods is the ease of use of the software used to run the simulations.
Mirroring the shift that was seen in synthetic approaches, the application of free energy calculations has moved in recent years beyond its starting point of carefully overseen and hand-tweaked calculations on individual molecules to a much more industrialised approach. Large numbers of candidates for synthesis are routinely triaged computationally to narrow chemical libraries down to the desirable regions of chemistry space. The overall hunt for a clinical candidate has been made significantly more efficient, with each cycle in the process consisting of the synthesis of a library of compounds pre-screened for high binding to their target.
One application of free energy methods that has yet to reach its full potential is the synergy with AI-based generative chemistry. Generative chemistry offers a step change in how we approach drug discovery, with the promise that an AI can learn the underlying features behind protein-ligand binding and hence design better molecules than a human expert. Similarly, advances in AI protein-ligand co-folding models may remove the requirement for good-quality experimental structural biology data. However, there is some debate about whether current models truly do manage to learn chemistry or whether they just memorise their inputs.8
In the meantime, generative chemistry methods are widely used but are generally inaccurate, such that post-scoring of the generated molecules is necessary. Free energy methods would be ideal for this but, since molecules created by generative chemistry methods are usually structurally unrelated to each other, this needs to be done by much more computationally expensive and less accurate absolute binding free energy (ABFE) calculations, which do not require a congeneric series. Advances in computing power and improvements in the underlying methods will no doubt make the use of ABFE to triage and validate molecules created by generative chemistry a routine approach in the future.
An important factor in facilitating the widespread use of free energy methods is the ease of use of the software used to run the simulations. When calculations took days and required a large amount of specialist knowledge to run, it was perhaps acceptable for the software to be hard to drive and very complex.
However, in a world where it is expected that every candidate for synthesis should have been checked by a free energy calculation beforehand wherever possible, the requirement from users is that running the calculations should be as simple and as automated as possible.
In the Flare™ package from Cresset, for example, the setup of a free energy calculation is not only fully automated, but the software checks for and warns about a large number of possible failure modes and system features that can lead to less accurate results. This reduces the likelihood that a calculation can be run that could give erroneous or misleading results. This guided approach maximises the efficiency of experienced computational and medicinal chemists, while also ensuring that less experienced or knowledgeable users can feel confident that the experiment they are undertaking will be accurate. Just as important is the software’s ability to easily access computing resources in the cloud, as many organisations do not have direct internal access to the computing resources required to run free energy calculations at scale.
With mainstream adoption of free energy methods across pharma and biotech, drug hunters are further pushing its boundaries and ever expanding its domain of applicability. Software that is easy to use while being powerful and scalable is helping to drive this progress.
Free energy perturbation (FEP) software supports scientists in making known actives more potent, without having to synthesise hundreds or thousands of compounds, eliminating the time wasted on synthesizing non-potent molecules. How can you accelerate drug discovery using FEP calculations?
References
1. RW Zanwig, J Chem Phys, 1954, DOI: 10.1063/1.1740409
2. C de Oliveira et al, J Chem Theory Comput, 2019, DOI: 10.1021/acs.jctc.8b00826
3. M Papadourakis et al, J Chem Theory Comput, 2024, DOI: 10.1021/acs.jctc.4c00942
4. F Zariquiey et al, J Chem Inf Model, 2023, DOI: 10.1021/acs.jcim.3c00178
5. B Ries et al, J Comput Aided Mol Des, 2022, DOI: 10.1007/s10822-021-00436-z
6. V Gapsys et al, Commun Chem, 2021, DOI: 10.1038/s42004-021-00498-y
7. CJ Woods et al, J Chem Phys, 2024, DOI: 10.1063/5.0200458
8. P Škrinjar et al, bioRxiv, 2025, DOI: 10.1101/2025.02.03.636309
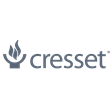
No comments yet