More organisations are moving away from traditional trial and error methods and adopting design of experiments to drive rapid innovation
Gaining and maintaining competitive advantage requires today’s organisations to innovate at increasing speed. As products and processes become more complex, subject matter expertise alone does not always deliver progress at the pace demanded. ‘Trial and error’ methods do not always yield optimal solutions when time is limited. A more systematic approach is required.
Watch the panel discussion and case study to find out how design of experiments can solve intractable problems and create novel products.
Many experts working in R&D rely on a traditional approach to this problem, changing one variable at a time. It might seem to offer the structure for more predictability but such an approach can end up constraining innovation and decreasing efficiency in the long run.
Peter Goos, professor at the Faculty of Bio-Science Engineering of the University of Leuven and the Faculty of Applied Economics of the University of Antwerp, explains the shortcomings of relying on ‘one factor at a time’ methods: ‘[It] overlooks synergistic and antagonistic interactions. It does not use proper randomisation, and lurking variables might spoil results and lead to false conclusions.’
The answer for an increasing number of organisations is design of experiments (DOE). DOE enables you to look at interactions, utilise randomisation and change multiple factors – all leading to a better understanding of processes with fewer experiments. Proponents of DOE claim significant advantages, so why aren’t more scientists seizing the opportunity to optimise processes while saving time and money through adoption of designed experiments?
As humans, we struggle to visualise a multivariate experimental space
Pilar Gomez Jimenez, Johnson Matthey
Overcoming objections to DOE
Pilar Gomez Jimenez, principal scientist at Johnson Matthey, has a master’s degree and a PhD in chemical engineering. She has been working in R&D of catalysts and materials for over 15 years and is an enthusiastic practitioner of DOE.
At Johnson Matthey, DOE has delivered 50–70% savings in time and resources. She says that gaining management support has not been a problem. According to Gomez Jimenez, the main obstacle when encouraging others to adopt DOE is always the human factor: ‘As humans, we struggle to visualise a multivariate experimental space. We are able to imagine the effect of changing temperature, but if we are moving temperature and pressure at the same time, then analysing the data gets more complicated and our minds struggle to visualise it.’
Perhaps because many scientists are taught to work with one factor at a time or because they have been doing things a certain way for a long time, it can be difficult to persuade teams to overcome their initial fear of statistical approaches to experimentation. Gomez Jimenez says it is essential to support colleagues to explore something new, particularly where a statistical approach is required.
Seeing the transformational results of DOE first-hand is often an excellent motivator for organisations to embrace a new approach
Widespread adoption of DOE can often be hampered by initial fears that the process will be onerous, or that the scale and type of problem is not suited to DOE. In fact, DOE is a methodology that scales regardless of the size of the problem. It provides the ability to thoroughly explore the opportunity space, enabling scientists to define an efficient and effective data collection plan that enables them to build a model of the product or process. That model can then increase understanding of the problem, drive decisions and enable consensus with other stakeholders. The more complex a problem and the more factors involved, the bigger the opportunity space to be explored – and the greater the impact of DOE.
Achieving life-changing results
Tim Gardner is a scientist and the CEO and founder of Riffyn, an organisation enabling scientists to easily combine DOE with efficient data management and contextualisation. Gardner said recently that DOE has changed his life, just as it has changed organisations where he worked in the past. Some plants where he worked with DOE experienced a productivity boost of 10–15%. Time to market for new products reduced fourfold, sometimes more, in one case shrinking from 15 months to three months.
Results like this inspired Gardner to found Riffyn and seek to make DOE a standard way of operating for more organisations. But he recognises that people fear change and for many DOE represents the unknown. Whether there is confusion over the term DOE and what it actually means, or uncertainty over the use of maths and statistics, fear can prevent many organisations from seizing the opportunity. Seeing the transformational results of DOE first-hand is often an excellent motivator for organisations to embrace a new approach.
DOE helps scientists design and analyse results rapidly and in a visual way, enabling them to understand analyses quickly and explain them to other people. Visualisation is key to demonstrating the value of the process to the wider organisation without needing an in-depth knowledge of statistical analysis. Whether supporting the delivery of better solutions, products or services, a statistically designed approach to experiments can accelerate the discovery and creation of viable new solutions and ensure that they can be delivered at pace and at scale.
DOE offers plenty of advantages beyond saving time and resources. Improved predictability helps with planning and enables more efficient ways of working
Vicky Svidenko, who leads the quantum systems integration team at Microsoft, describes adopting DOE as having a ‘snowball effect’ on her organisation. Microsoft’s culture encourages people to look for opportunities to experiment and celebrates acquired learning.
DOE offers plenty of advantages beyond saving time and resources. Improved predictability helps with planning and enables more efficient ways of working. This efficiency can help to ease the stress levels within teams under pressure to deliver. Organisations can make better decisions faster and meet project milestones more predictably. Reducing the stress on individuals by giving them the right tools to be productive and efficient can benefit the whole organisation.
Creating the time and space for innovation
By actively manipulating factors according to a pre-specified plan, it is possible to gain a new understanding of relationships between inputs and outputs; achieve faster, more predictable cycles; and save time: in short, to know when a concept will work and when it will not.
Gomez Jimenez explains that because ‘you’re looking at the full experimental space and getting a proper map of what the results will be and the interactions between the variables, the result is greater insight and confidence in the conclusions.’
With the right tools, it is easy to get started and experience some quick wins, which in turn helps to convince colleagues, teams and the wider organisation that there are significant benefits to be achieved with DOE. Bradley Jones, senior research fellow at JMP, calls DOE ‘active learning’ and highlights how it gives organisations the ability to ‘fail fast’, which in turn drives rapid innovation. Perhaps reframing DOE in these terms would help to demystify it and encourage more scientists and organisations to make the leap.
Watch the panel discussion and case study to find out how design of experiments can solve intractable problems and create novel products.
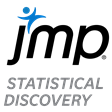
No comments yet