A new machine learning tool for drug design proposes better and more realistic drugs through clever handling of molecular geometries. The FragGen software builds molecules fragment by fragment, and uses different machine learning processes for each decision, to minimise the inherent drawbacks of each. FragGen’s creators were able to select an anticancer target, design a new drug, synthesise it and demonstrate its potency experimentally.
Many drugs work by binding to a particular pocket in a protein. A drug that binds strongly to its target will tend to be more potent. Machine learning methods should be able to learn the relationships between the structures of these targets and the qualities of molecules that are good at binding to them. However, the number of examples available for teaching models is small in comparison to the space of all molecules and all protein pockets, so a drug-inventing oracle isn’t yet on the cards. Some models might propose chemically unreasonable arrangements of atoms, while others might suggest molecules with lots of fused aromatic rings, which are chemically plausible, strongly binding, but un-drug-like and hard to synthesise.
A chemically sensible structure can be made more likely by building the molecule piece by piece from fragments. ‘Previous structure-based molecular generation methods basically learn how to tightly bind to the molecules, often learning what the real, synthesisable, molecules look like implicitly through training,’ explains Odin Zhang of Zhejiang University in China, one of FragGen’s creators. ‘Designing molecules based on building blocks forces the molecular generation path to be synthesisable.’ However, the stepwise nature of this approach means any error in the placement of each fragment will accumulate, so the molecule could be twisted into an implausible geometry in the binding pocket.
FragGen is a fragment-based method that imposes order by handling the molecule’s geometry in a very deliberate way. Starting from an empty pocket, one machine learning model decides which atom a new fragment should be added to; another what that fragment should be; another how it should bond to the target atom; and so on for geometric variables like bond and dihedral angles. It addresses each of these smaller problems with a different model known to be good for that kind of prediction.
Breaking the problem down in to a series of steps that can be addressed by simpler models is ‘a really good way of devising scientific tools that use deep learning in a thoughtful manner,’ says Ré Mansbach, a computational biophysicist at Concordia University in Canada. ‘I think people get really excited about the idea that you can just throw the data in and it comes up with some model, but I don’t think it’s necessarily the most effective way to do it’.
FragGen’s drug suggestions score highly on a number of common theoretical measures for potency and ease of synthesis. More importantly, they pass muster in experimental tests. The researchers used FragGen to design a type-II kinase inhibitor. The software suggested 97 candidates in just 10 minutes, the three most favourable ones were found to be both synthesisable and effective at micromolar and nanomolar concentrations.
To be safe, a drug must also be specific to the binding pocket, and not also bind to and influence other important proteins. Tools such as FragGen can’t yet do this, but they offer a valuable starting point in the discovery process. ‘Where you want to apply artificial intelligence and deep learning approaches is usually right at the beginning of design, so that you can scan the space for things that you haven’t thought of and narrow your search down to something manageable,’ says Mansbach.
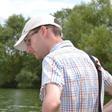
No comments yet