There’s nothing like the automation of chemical synthesis to get chemists worried. Could robots and artificial intelligence (AI) one day replace them, just as they seem to threaten so many other activities and jobs? A project led by a team at the Massachusetts Institute of Technology (MIT) seems now to imply that the answer could be yes – and no.
Klavs Jensen and his co-workers have unveiled a system in which an AI algorithm devises and refines a synthetic strategy for target molecules, and a robotically controlled experimental platform then carries it out.1 But crucially, the scheme still relies on some expert human judgement for success. The best way forward, the work suggests, is to play to the strengths of both machines and people.
The approach is really about ‘how we can aggregate our collective knowledge of chemistry and use that to design synthetic processes to make new molecules more easily,’ says MIT team member Connor Coley, who worked on the system for his PhD project. Jensen explains that the system ‘is meant as a tool that will enable us to more quickly make molecules for what we’re potentially interested in doing with them’.
The idea of using AI to plan chemical synthesis has been around for some time.2 For example, one team of researchers recently showed how a ‘deep’ neural network, which uses several layers of processing nodes, can devise efficient retrosynthetic routes to molecules that experts judged to be as good as those planned by humans.3 Typically such algorithms are ‘trained’ using vast numbers of published syntheses, so that they can apply similar strategies to make compounds outside the training set.
At the same time, researchers are interested in automating laboratory experiments, for example using robotic manipulators, to speed up the rate at which syntheses can be tried and evaluated.4,5 These two developments might ultimately be combined so that synthesis can be both planned and conducted without human intervention. However, so far such robotic schemes often need human help, for example to configure the apparatus for a particular synthesis, and are not easy to scale-up.
AI, robot
The system devised by Jensen and colleagues overcomes some of these limitations. They use an AI scheme to plan and assess the synthetic route, and the machine’s recommendations are then enacted by a robotic system, which autonomously puts together standardised microfluidic components and reagents to run the synthesis using continuous-flow chemistry.
‘This is the first example of the integration of all the elements: retrosynthesis, identification of reaction conditions, and prediction of whether the reaction is successful,’ says Jensen – as well as its automated implementation by a robotic system. ‘I don’t believe there has been a previous robot that is capable of configuring a flow chemistry system and then executing the reaction,’ he adds. ‘Once the recipe is done, all you do is press the button and watch the robot do everything else.’
However, human help is still essential. ‘The data is not sufficient to make a prediction of the full recipe by AI, including concentrations and so forth’, Jensen explains. ‘You can partially plan it but you need an expert chemist to finish it.’ For example, he says, ‘sometimes we changed the conditions that came from the program, or we changed one particular reactant – replacing a chloride with a bromide to improve solubility, say’.
What’s more, the human expert’s input was often needed to translate results in the literature for ‘batch’ processing, where the reaction stoichiometry is controlled by concentrations and volumes of reagents, to flow processing, where it depends on flow rates rather than volumes. ‘There’s a still a very important degree of the chemist’s involvement,’ says Coley.
Breaking synthesis down
To make a given target molecule, the algorithm applies the standard approach called retrosynthesis, which systematically breaks down the target into progressively simpler compounds by cleaving bonds that can be constructed using known chemical reactions. The algorithm draws on a database of 12.5 million single-step reactions, which are boiled down to around 164,000 reliable rules. Each suggested step of the retrosynthesis is evaluated by a neural network trained on 15 million examples that are known to work, along with 115 million that are predicted not to. In each case the system asks whether there is any set of conditions for which the given reactants will form the intended product. The ultimate goal is to reduce the target compound to small molecules that are readily and cheaply available from suppliers such as Sigma-Aldrich.
To test their scheme, Jensen and colleagues chose 15 small target molecules of medical relevance for which synthetic routes already exist, from aspirin to diazepam (Valium). They also selected some targets containing challenging chiral centres, such as the anti-coagulant (S)-warfarin and safinamide, used to treat Parkinson’s disease. In all cases the system came up with strategies that gave acceptable product yields at relatively low prices. The products are purified ‘offline’ using methods such as high performance liquid chromatography, but Jensen says this could be added into the automated system.
One of the key current limitations of the approach is that the flow system can’t cope with solid-state processes or products. Lee Cronin at the University of Glasgow, who was not involved in the work but is also working on AI systems for automated synthesis, thinks that the cost of the equipment might also be restrictive.
But Cronin adds that ‘the key and important thing here is the integration between the use of AI and robotic chemistry’. Although the system doesn’t seem ready yet for applications such as drug discovery, he says, ‘the point is to have a robot that can access on the fly a finite but important set of molecules, and in this sense the work is impressive’.
Synthetic chemists still wanted
Jensen says that if there was more and better data available, for example on reaction conditions, more of the synthesis design could be done without human intervention. ‘It would be possible for the AI to learn what the conditions should be, so that a larger portion of the recipe file could be finalised [by the AI]’, he says.
So far the system can only use reactions already known. But there’s no reason to suppose that these capture all of the best ways to make a given bond, and the researchers hope that AI might eventually be used to predict entirely new synthetic steps, based on the outcomes of those already known. The team is now looking at ‘whether this [approach] can be extrapolated to entirely new ways of making molecules’, says Coley.
But Jensen cautions that ‘it would be very difficult to build a general purpose synthesis that could build any molecule’. ‘Chemical space is huge and there are many molecules that the best expert synthetic chemists, with all of the tools at their disposal, would still have a hard time synthesising,’ Coley adds. ‘I don’t think it is realistic to say that we’ll reach a point where these automated systems will be able to synthesise the same scope of molecules as the best human chemists. But there might be smaller chemical spaces that are easier to access.’
So systems like this will be assistants, not replacements. ‘If you have systems that can make the simple stuff, then you can begin to explore other parts of the space that we so far haven’t been able to explore because it takes us so long to get what we need to get there,’ says Jensen.
At any rate, the human chemist is not likely to be replaced any time soon. ‘These machines don’t discover,’ says Jensen. ‘If you’re going to discover new molecules in the laboratory, machines may help you to do some of the things – but you still have to have understanding of how you manipulate the chemistry.’
References
1 C W Coley et al, Science, 2019, 365, eaax1566 (DOI: 10.1126/science.aax1566)
2 S Szymkuc et al, Angew. Chem., Int. Ed., 2016, 55, 5904 (DOI: 10.1002/anie.201506101)
3 M H S Segler, M Preuss and M P Waller, Nature, 2018, 555, 604 (DOI: 10.1038/nature25978)
4 G Schneider, Nat. Rev. Drug Discov., 2018, 17, 97 (DOI: 10.1038/nrd.2017.232)
5 S V Ley et al, Angew. Chem., Int. Ed., 2015, 54, 3449 (DOI: 10.1002/anie.201410744)
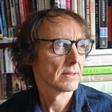
No comments yet