Reticular chemistry pioneer Omar Yaghi has co-founded a new institute focused on using AI to tackle climate challenges. He spoke to Chemistry World about what the Bakar Institute of Digital Materials for the Planet at the University of California, Berkeley aims to achieve and how AI will change the way we do chemistry.
Why is AI so attractive to reticular chemists?
AI has a transformative role. In reticular chemistry, the synthesis of materials like metal–organic frameworks (MOFs) and covalent organic frameworks (COFs) relies on well-established chemical conditions. We use AI to rapidly optimise the reaction conditions for various building blocks, to create new frameworks with the desired properties.
AI can help in predicting and mapping the vast space of potential structures that arise from different molecular geometries, significantly accelerating the identification of promising candidates and saving resources.
Reticular chemistry enables chemists to not only do the basic science but to engineer their product into a prototype to show the market how it will work for a specific application. AI is still in its infancy in terms of its application to science, but I feel we need to experiment with it. We need to engage with our computer science colleagues, to help them understand how we think and how discoveries are made in chemistry and to make sure that those models are improved to enhance chemistry.
How has AI helped your work?
MOFs have a metal ion and a charged linker, making a very strong bond. One linker we thought would be interesting is hydroxymate. Hydroxymate MOFs are difficult to crystallise and in five years we’ve made only one. By using a suite of large language models working together, we can mine texts and images in the literature to give us an idea about what direction we should go in to find conditions under which a specific set of building blocks are going to make a MOF or a COF. With these tools, one of my new students made 15 new compounds in just six months.
The next task is to predict its properties – will it be a great carbon capture material or be able to harvest water? We use chemical computation and quantum mechanical computation to try to understand the environment inside the pore. Alongside we’ve developed a machine learning algorithm that incorporates all the features we chemists think are important for the properties we’re looking for. And bit by bit you develop a mathematical framework that enables the algorithm to make better predictions than you would at random. Using our models, which we call Foundational Gen AI models, we’re doing 50% better than random.
How can these models get better?
This is systematic, rigorous work that documents the successes and failures, which is something that we chemists haven’t been doing, thus far. Knowing what doesn’t work improves the machine learning algorithms.
It’s hard for scientists to tell the world what didn’t work because it’s a reflection of how smart their design was. But I think journals and publishers can drive this, so what didn’t work becomes part of the literature.
Can AI help save the planet?
The discovery of new materials needed to address global challenges such as climate change is a slow process. It involves exploration of a huge chemical space and, without the aid of robotics and AI, our ability to find the appropriate material will take longer and use more resources. AI is essential to accessing materials faster, better and cheaper.
We can use the models to look for green building units and find out what structures they can make and under what conditions they’ll crystallise. Once we make them, we’ll know exactly what to do to make, for example, a carbon capture material based on what we’ve already learnt. I think that ultimately is where chemistry is going.
By accelerating the discovery of sustainable materials, AI can drive innovations in clean energy, efficient storage and carbon capture technologies. These advances reduce greenhouse gas emissions, promote renewable energy adoption and minimise resource waste.
What would your advice be to students on AI?
AI is absolutely essential for the future of chemistry. I have been telling incoming students that every student in my lab will have to learn how to use AI to facilitate and speed up chemical research. We would be doing them a disservice to allow them to continue in the old-fashioned way. To prepare the next generation to think outside the box, to compete with other fields and solve the difficult problems in society, they’re going to have to be well versed not just in chemistry, but in engineering, and in using AI and computation.
What have you learnt from computer scientists?
That if it doesn’t scale it’s not worth looking at. Everybody needs to take advantage of your discovery and that’s ultimately how the world gets better. Part of scaling up is enabling everyone to access a platform for, say, making MOFs and COFs. You go to this platform, plug in questions and the platform comes back with potential MOFs and COFs that you could use. It guides you step by step to make and test them and potentially tells you who to partner with to scale up production. What that means is that chemistry will no longer be an exclusive club, but open to all.
This interview has been edited for clarity and brevity.
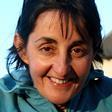
No comments yet