Combining spectroscopy and artificial intelligence (AI) data analysis has revealed new insights about the murals that were painted on the Berlin Wall. The new approach could provide high quality data for art conservation and restoration – identifying better ways to understand the techniques and materials used in street art.
The team used a handheld Raman device to identify pigments on paint chips from the Berlin Wall. As handheld devices lack precision and sensitivity compared with standard lab equipment, the researchers also used AI to improve the analysis by the portable devices.
The team first examined 15 paint chips and observed that all had a maximum of two or three layers of acrylic paint – brushstrokes as opposed to spray paint. Then, they used Raman spectroscopy to characterise the chips and identified titanium white, azopigments (yellow and red chips) and lead chromate (yellow) as the primary pigments present.
By mixing common, commercial paint with titanium white the scientists quantified the dye dilution in mixtures used by the artists. They trained a machine learning algorithm to predict the ratio between the pigments using Raman data at various pigment concentrations. From the dataset, they extracted wavenumber values as features while concentration values served as labels. Thus, a custom neural network was built for regression tasks, predicting pigment concentrations. It was discovered that the paint chips contained titanium white and up to 75% pigments, depending on the piece of wall from which it was sourced. The results rivalled those achieved using laboratory equipment.
The authors claim that the new AI can be a significant tool in preserving the murals of the Berlin Wall, which are vulnerable to degradation. The researchers hope that the synergy between AI and chemistry will transform Raman spectral analysis in the field across a range of disciplines such as forensics and material science.
The colour of lead chromate was corrected on 9 April 2025
References
F Armetta et al, J. Am. Chem. Soc., 2024, DOI: 10.1021/jacs.4c12611
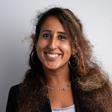
No comments yet