An artificial intelligence (AI), trained on the scientific literature, and combined with an automated chemistry platform has been used to both discover and synthesise inorganic compounds. The researchers hope that the AI can be used to speed up the discovery of novel materials such as recyclable plastics and transparent conductors for cheaper solar panels.
Discovering energetically favourable inorganic crystals is a fundamental focus of solid-state chemistry, but identifying promising new materials without automation can be challenging and time-consuming.
In the first of two papers on the topic, researchers at Google DeepMind developed a deep learning tool called Graph Networks for Materials Exploration (Gnome), which they trained on a large and diverse data set developed by the Materials Project – an open-access database created by the Department of Energy’s Lawrence Berkeley National Laboratory in 2011 – and used it to filter candidate structures.1
They showed that, by improving the Gnome algorithm through active learning, they were able to discover 2.2 million stable structures, including 381,000 new entries for the Materials Project. This, the researchers said, represented ‘an-order-of-magnitude expansion from all previous discoveries’.
‘At the beginning of this pipeline we were getting about 10% of the materials that we were looking for were actually stable,’ explains Amil Merchant, an AI resident at Google and lead researcher on the study. ‘By the end of training and by the end of these rounds of active learning this efficiency number was all the way to 80%.’
Some of the computations from Gnome were used alongside data from the Materials Project to test an autonomous laboratory (A-Lab) facility that was the focus of the second study.2
Automating inorganic chemistry
‘We were trying to build a lab that can run by itself,’ explains Yan Zeng, a scientist at Lawrence Berkeley National Laboratory in the US, and one of the researchers who developed A-Lab. ‘We’re focusing on one specific problem in materials science, that is, the synthesis of materials, and more specifically, we’re focusing on one particular synthesis method; the solid-state reaction, one of the most popular ways to make materials, especially inorganic materials.’
A-Lab uses historical data from the scientific literature, machine learning and active learning to plan and interpret the outcomes of experiments performed by robots. Over 17 days of independent operation, A-Lab performed 21 experiments a day and was able to produce 41 new compounds out of an attempted 58; a success rate of 71%.
‘This kind of solid-state synthesis usually takes a human one or two days for one to two experiments … the whole process is pretty tedious, it takes a lot of time in the lab and it also takes a lot of time to analyse the data once the data is measured if it’s done manually – so here the efficiency is really boosted, around 50 to 100 times higher,’ explains Zeng.
Zeng also highlighted that the A-Lab had high levels of precision, compared with a human, enabling it to repeat the same experiment repeatedly with a much lower error rate and was able to learn from past data to correct failures through a closed loop system. The researchers said that A-Lab’s success rate could reach almost 80% with minor modifications to the decision-making algorithm and improvements to the computational techniques used.
‘These two papers together represent a very important step forward in our ability to predict stable materials and then transform them into physical form in the laboratory, and I think it’s a powerful one–two punch, which really moves the needle in that important space,’ says Martin Burke, a chemist at the University of Illinois Urbana–Champaign in the US, who has an interest in automation.
However, he says an important limitation with the two studies is that they do not yield new knowledge. ‘A really important question is how to turn the power of that marriage between AI and automated synthesis into discovery engines to find new functions and to find new knowledge; that’s going to be an exciting question moving forward.’
Lee Cronin, regius chair of chemistry at the University of Glasgow, says the two papers are ‘certainly an advance’. ‘It’s proof that if you have a clean workflow you can generate new experimental ideas, which are consistent. The papers are obviously high quality [and give] more momentum to the idea that AI and clean data can be used.’
‘But I’m interested in how we would go further. There’s a danger people will stop doing experiments and just keep simulating things … we need to look where the anomalies are – a lot of excitement is going to happen when we can use these to discover anomalies, unexpected things.’
References
1 A Merchant et al, Nature, 2023, DOI: 10.1038/s41586-023-06735-9
2 NJ Szymanski et al, Nature, 2023, DOI: 10.1038/s41586-023-06734-w
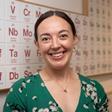
No comments yet