What can chemists learn from ideas in economics? Daniel Kahneman, who died in March this year, was a psychologist who won the Nobel memorial prize in economics in 2002 and I still remember how his book Thinking Fast And Slow affected my career. It made a big impact on me when I was just beginning to understand the power of statistical thinking.
The book tells the story of Kahneman’s own career, including his collaboration with fellow psychologist Amos Tversky (who died in 1996) and their work uncovering surprising flaws in human decision-making that would give birth to behavioural economics and earn him a Nobel prize. It explains the imperfect heuristics or short-cuts we use for ‘fast’ thinking and how many of our choices are not purely ‘slow’, deliberate, rational thinking. And that this can lead to costly decisions. In a complex world of increasingly abundant data and the powerful potential of probabilistic machinery of AI, the lessons of Kahneman and Tversky’s research are more relevant than ever.
Slow down
In one of Kahneman’s examples, hundreds of millions of dollars were spent to make US schools smaller. Someone looking at the data noticed that the top 50 schools included an unexpectedly large number of small schools. Conclusion: let’s break up big schools to improve their performance.
Unfortunately, they didn’t look at the 50 worst schools, many of which were also tiny! This is what Kahneman calls ‘the law of small numbers’: we fail to appreciate that averages of small samples are less reliable and yield more variable outcomes. In this case, the smaller the cohort of students, the more likely it is for them to be exceptional – in both good and bad senses. A careful analysis of the data shows that students do better in larger schools, on average, with more course options and resources.
Chemists tend to seriously underestimate noise in their data
We can be more critical when we recognise biases like this. I was sceptical when I heard that a national annual survey had found my home district of Craven in North Yorkshire was the happiest in the country. It’s beautiful here, but it also rains a lot. I dug into the study and was not surprised to find only a few dozen people are surveyed in Craven each year, compared with hundreds of respondents in urban areas. Looking back over many years, the ‘best’ and ‘worst’ areas each time are always sparsely populated parts of the country with wildly variable estimates of life satisfaction due to small sample size.
Short-cut chemistry
Chemists can also be prone to some ‘fast-thinking’ flaws in problem-solving where a ‘slow’ statistical mindset would be better. Chemistry is taught primarily as a deterministic system – when you know where the curly arrows are going, you know exactly what will happen. Yet that also means chemists tend to seriously underestimate noise in their data, if they consider it at all. That’s a problem when trying to unambiguously find the important signal that will take you to the solution. Thinking statistically means having to understand the variation in real-world systems.
Chemists are also given rules-of-thumb that can be a barrier to applying statistical thinking: Reaction rates double with every 10 degrees; “when MW is below 200 amu, beware of rotoevaporation.” These are useful shortcuts but they imply a simplistic rules-based order to chemistry, when the reality is often much more complex and probabilistic.
We are also encouraged to develop intuitions and fast-thinking habits that we can’t set out as rules. We might describe this chemical intuition as ‘tacit knowledge’, a concept from another economist and philosopher, Michael Polanyi. Polanyi also made important contributions to chemistry and although he was never awarded a Nobel Prize himself (he was nominated for his work in both physics and chemistry), two of his pupils and one of his children did become Nobel laureates.
Humans and AI can both suffer from availability bias
Tacit knowledge is that which we know, but can’t say why we know. This differs from the explicit knowledge that can be written in a text book or as a set of instructions. Polanyi said ‘we can know more than we can tell.’ You can imagine this to be true of an experienced synthetic chemist with a reliable sense of what routes and conditions are most likely to be successful. Kahneman was initially dismissive of the value of ‘expert judgement’ like this but later accepted that intuition can be trusted when it is learned in an environment that is sufficiently regular with close feedback loops.
There are important consequences here for how we work alongside AI. We will need a chemist-machine partnership to augment the explicit knowledge that can be captured by data models and Kahneman’s model helps us to understand the power and limitations of AI. AI can ‘think slow’, without many of our biases, using vast computation power to do this relatively quickly. However, humans and AI are sometimes more alike: we can both suffer from availability bias where we rely disproportionately on the most readily available data.
Statistically speaking
I wonder if there is more that we can do to bridge the gap. I recently talked with Markus Gershater, chief scientific officer of Synthace, for a new podcast series. We discussed how organisations might get value from aggregating their experimental data across multiple projects. It seems unrealistic that you could ever use that data to replace real experiments – the possibility spaces we are exploring are too vast. But, where there are sufficient similarities from one project to the next (Gershater gave the example of biological assay development) then data models could provide the kind of guidance that you might get from a scientist that has been doing these things for many years. A data model can’t tell you the optimum conditions for the next assay, but it might tell you the ranges of temperature, pH and reagent concentrations that you should explore.
As ever, data quality is paramount. Well thought-out, structured experiments will provide the best data for training AI and minimise problems due to our human biases. Maybe we also need to think about how we introduce concepts of variation in our scientific education. Being made to calculate ‘error’ feels like a punishment for not getting the exact same result from three titrations and likely prevents us from embracing uncertainty in our scientific endeavours. Instead, examples from the real world of industrial problem solving can help to prove the value of learning to think slow and statistical.
You can follow #DOEbyPhilKay on LinkedIn for my weekly posts on Design of Experiments and for breaking news on the upcoming podcast series.
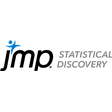
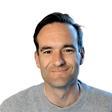
No comments yet