In the 18 months since OpenAI launched Chat-GPT, generative AI has boomed. The latest iteration, Chat-GPT-4o, launched in May this year and in the meantime the other big tech players have all released their own contenders. As these companies compete to develop and deliver better versions of their algorithms, they are pushing the limits of what’s possible, but also the limits of what’s available: they are running out of data.
To train the next generations they need new and bigger datasets of human language and knowledge, and even the billions of words in literature and the internet are no longer enough to satisfy their impatient appetites. A solution being explored by many companies is therefore to have their polyphagic progeny feed themselves: machine models can generate datasets to train other machines – an ‘infinite data generation engine’ as one tech company chief executive put it recently.
The idea of dummy data built from real data is not new – it’s used to generate anonymised datasets in healthcare, for example – but building an infinite data generator takes things to a new level. As we explore in our feature article, it’s also an idea that’s being used in chemistry.
The promise of synthetic data is that it could not only supply more data more quickly, but the right kind of data
The experts in our feature note that the idea of synthetic data might seem unscientific at first glance. But chemistry already uses plenty of data that didn’t come directly from ‘real’ experiments. We’ve known how to model atoms and molecules for over 100 years and for the last 60 or so we’ve had the tools to implement that knowledge in computer programs. Large databases of molecules have been built by simulating those systems in a computer, which in turn have been used to train machine learning models. The difference here is that these models start with a formal theoretical description, rather than just empirical analysis of the data.
The downside with these physics-based approaches is that producing enormous datasets still takes a lot of time and speeding them up means compromising their quality. Atomistic simulations of this kind are also only suited to particular kinds of problems. The promise of synthetic data is that it could not only supply more data more quickly, but the right kind of data; the examples in our feature show there are many problems where it can help. And there are also those where it won’t.
A recent review on the future of AI in retrosynthesis highlighted the dearth of negative results and the low diversity of reactions in the literature as key barriers to making retrosynthesis algorithms more useful. These models are only as good as the datasets used to make them and those that rely on the scientific record are particularly prone to the creeping bias in that dataset towards positive results, which gives them a skewed perception of reality. And chemists are not known for being adventurous when it comes to reaction choice, either.
To improve those algorithms, they need to know what doesn’t work, as well as what does. That sort of data does exist, but generally only as painful memories in the minds of chemists or in the discarded notebooks and dusty drawers where unpublishable results go to die. Synthetic data approaches have been trialled to artificially augment such datasets, but with limited success. And in a note of caution, a computational science preprint published last year showed theoretically that in recursive loops where models train other models, the system’s underlying truth can be ‘forgotten’.
The solution proposed by the authors of the AI retrosynthesis paper is instead to make better use of human expertise. Using our existing knowledge to target the lacunae of the literature will deliver progress much more quickly than waiting for campaigns of computational or real world experiments to deliver more data. This echoes the general principle of keeping humans in the loop that is advocated by many groups developing AI-assisted work of all kinds. Collaborations between human and artificial intelligences are not simply about the division of labour, but ensuring that human agency and responsibility are preserved in the process.
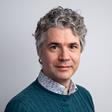
No comments yet