Creating a purpose-built repository of standardised reaction data is a tall order, but the reward would be huge
I have a small problem with how the chemistry community handles data, and it comes up whenever I go hunting in the literature. For example, I often look for process optimisation case studies showing the value of statistical design of experiments, or for repositories of material properties that I can use to illustrate the power of machine learning. And I often find them: more and more studies using data-driven methods are being published and more authors and publishers are making the associated data available.
But actually getting my hands on the data is not always so easy. It might be in a table in the article, or more often in a separate pdf. Or it might be in a spreadsheet file, or a more exotic format like JavaScript Object Notation (JSON). Sometimes it’s in a zip file and there might be associated Python code. In the worst cases, it’s just an image.
The goal should be to create the best possible data repository for chemistry AI
For me, this lack of standardisation is an inconvenience. But for chemistry, it’s more serious because we’re missing out on all of standardisation’s benefits. And that’s not just boosting efficiency and collaboration – ISO standards for laboratory practices in pharmaceuticals, for example, are critical for patient safety. To harness the potential of chemistry data, we need standardised descriptions, but we also need to design them based on what we want to achieve.
Fair play
One of the most successful efforts here has been in analytical chemistry, and especially chromatography. The Allotrope Foundation, for example, is a collaboration between research organisations and companies, including competing hardware providers, that has created a standardised format for analytical chemistry data. It describes the experimental parameters, processes and results, and connects them with metadata on people, places, equipment and studies for context. More generally, there is the Fair initiative that promotes making research data Findable, Accessible, Interoperable and Reusable. The International Union of Pure and Applied Chemistry is now leading the application of Fair principles in chemistry’s digital standards.
Yet despite this progress, the ultimate goal should not be to make all types of chemistry data Fair – this is neither feasible nor desirable. Instead, we should focus less on the data itself, and more on what we can do with the data. And given that one of the best uses we have for data today is to enable AI, surely the goal now should be to help create the best possible collective data repository for training chemistry AI?
AI for all
Researchers are already working on building such datasets from the existing literature, which represents a huge potential mine of data. A recent pre-print uses vision-language AI models to extract data from figures and tables in pdfs, for example. Yet those datasets will always be limited because they are incomplete – not least because of the absence of failed reactions.
What we need is to systematically generate a purpose-built dataset to feed the AI solution we want. This is the statistical design of experiments (DOE) approach, but on a much bigger scale. We would need high-throughput, fully automated experimentation and analysis to efficiently cover the vast possibility space. This approach is digital by design so everything from the experiments to the results and metadata will be immediately in the structured, machine-readable form we need. Protocols and results can easily be distributed for transparency and to share the labour and the fruits. Collaboration will be essential, given the scale of the challenge.
This could provide employment and scientific opportunities away from the current hot spots of R&D
Initially, the scope would have to be narrow – probably the kind of reaction condition screening that is done in early phase pharmaceutical development. The scope can broaden as hardware capabilities improve – the work itself would also incentivise those innovations – and the AI model would become more generally useful.
The creators of chemistry AI training data will need to be fairly rewarded and incentivised, and the work should also be shared in such a way that multiple automated chemistry labs around the world can contribute. This could provide employment and scientific entrepreneurial opportunities away from the current hot spots of chemistry and pharma R&D and be a catalyst for more innovation.
A well-resourced ‘big tech’ company might seem the obvious choice as an owner. But balancing commercial ambitions and transparency is hard. Google DeepMind initially did not disclose the code of its latest protein structure prediction model, Alphafold3 – apparently to protect its commercial interests – and indeed open-source copies quickly appeared when the code was eventually released. A better model might be funding from a consortium of pharmaceutical and chemical companies that would have the incentive of access for commercial use. Public research funding should be included, ensuring it is open for academic and non-profit researchers.
A few years ago I visited Basecamp Research, a biotech company in London. Their aim is to create a high-quality genetic dataset to train the next generation of AI models for solving biological problems. Part of Basecamp’s success is its partnerships with scientists and governments around the world that enable it to collect the physical samples of biodiversity used to build the dataset. Their model ensures fair and equitable benefit sharing and incentivises long term rewards including building the skills base and facilities for participation in the bioeconomy.
What I’m proposing here is a huge piece of work. It’s not going to happen without broad understanding of the need for this data. I think more chemists would intrinsically get it if they at least knew how to build models from data at the smaller scale, and this whitepaper from JMP is a great starting point on using data to support innovation. The same principles that power those examples across different sectors and companies, could be used to build a tool that would be transformative for chemists everywhere.
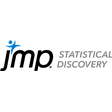
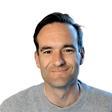
No comments yet