Statistical analysis can accelerate development, reduce costs and increase manufacturing quality
Competition and increased demand for product innovation are placing unprecedented pressures on chemical manufacturing. As well as a seemingly unquenchable need for new products and product variants, the industry as a whole is also burdened with the high cost of research and development. Though statistical analysis has not always gone hand-in-hand with chemical development, it can be a vital tool for accelerating the discovery and creation of viable new products, and for engineering the processes through which they can be delivered at scale.
Experimentation has always been a key aspect of product development, allowing the kinks in chemical and formulation processes to be ironed out. The traditional one-factorat-a-time approach to experimentation is partly responsible for inefficient product and process development: as well as consuming a lot of resources, it is likely to miss some of the practically important effects that lead directly to later manufacturing inefficiencies and failed product launches.
Fortunately, we now have the tools to supercharge our approach to experimentation. This method is well-established in many industries. By deploying this approach throughout the development phase, it is now possible to design quality into the process at the outset, rather than suffering the impact of failed product launches, protracted time to market, and low manufacturing yields.
Designing experiments to gather useful data
Design of experiments (DOE) is a systematic method to determine the relationship between factors affecting a process and the output of that process. There are usually many factors that might have an effect, and it is crucial that they be manipulated together, not one at a time.
DOE has been used to find cause-and effect relationships since UK statistician and geneticist Ronald Fisher first introduced it in 1935 and has been evolving ever since. This has led to a series of design families adapted to meet specific situations, and to more modern approaches that allow you to make a design that fits more or less any situation. Software tools like JMP do all the computing work, and make it relatively straightforward for chemists, researchers and engineers to easily adopt this new approach to experimentation.
The best way to gain useful new information
In statistics and machine learning, dimension reduction is the process of reducing the number of variables by obtaining a set of principal variables that still contain most of the information. While this technique has not traditionally been a part of the product development and testing process, it can be especially useful in conjunction with DOE.
In drug development, for example, many of the new products in R&D use starting materials that come from human beings or other animal subjects, and there is often only a short list of qualified donors. Thanks to advances in genomics and the characterisation of the microbiome, it is easy to generate a long list of measured properties from each of those subjects. This results in a very large set of measurements on a very small number of subjects. Testing this in the lab can be costly and time-consuming, but by using dimension reduction the analysis process can be streamlined.
Using statistics in R&D and testing
In a real example, 10 years ago a team making vaccines needed to identify what was causing some unanticipated results in manufacturing. They were faced with the challenge of evaluating around 1500 parameters affecting nine key quality attributes, measured on a few manufactured lots. The team included a chemometrician, a mathematician and a number of statisticians who, after several weeks of analysis, were able to recommend the changes needed to safeguard the supply of the vaccine in question. The computational challenges were tackled by a large team of people working tirelessly over several weeks and at great cost: today, using JMP, the same analysis problem can be solved by a single researcher in just 30 minutes.
A question of will (and training)
Appropriate training and know-how remain a big stumbling block for industry as a whole. Many chemists aren’t necessarily equipped to work with statistics, have not been exposed to DOE, and may not have had the opportunity to work with software like JMP that can give them the support that they need.
Promisingly, it is not uncommon to hear from scientists who were once reluctant to use DOE but who become firm advocates once they see how quickly they can find solutions for problems they had been working on.
The tides are shifting towards DOE
The wide adoption of DOE in R&D by the chemical-based industries should be seen as both necessary and strategic. Companies in these sectors should be actively investing in developing their DOE capability, and developing more comprehensive data collection schemes that allow them to better understand their processes through the whole product lifecycle. It is an effort in terms of training and investment, but it will allow them to survive and prosper.
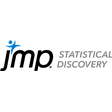
No comments yet