The Hammett equation, a chemical theory that is over 80 years old, is being expanded upon and improved with the help of machine learning. The equation, which can help to explain the electron-donating or withdrawing nature of aromatic substituents via calculation of Hammett constants, has been analysed computationally by a team of Brazilian researchers who want to make it even more precise, and unlock unknown values for practical experiments.
‘There are some experimental Hammett’s constants which, although widely used in many applications, were not measured or have inconsistent values,’ says Itamar Borges Jr from the Institute of Military Engineering in Brazil who worked on the study alongside Julio Cesar Duarte and Gabriel Monteiro-de-Castro. He adds that the work ‘employ[s] machine learning algorithms and available experimental values to produce a consistent set of the different types of Hammett’s constants’.
In 1937, Louis Hammett published work that led to the eponymous Hammett equation. He was working at the time in a new field that he had named ‘physical organic chemistry’. Hammett recognised the relationship between the rate of hydrolysis of a series of ethyl esters and the subsequent equilibrium position of the ionisation of the corresponding acids in water. It was some of the first work of its kind to try to provide a quantitative theory to rationalise the relationships between chemical structures and reactivity in chemistry.
Applying his focus to meta and para -substituted benzoic acids and their respective esters Hammett found a direct relationship. Each substituent on the respective aromatic ring could be given a value representing their electron-donating or withdrawing effect. These σ values were calculated experimentally by Hammett, helping chemists to determine the impact on reactivity from these groups. Hammett then went even further, deriving ρ values. These values meant chemists could predict the number of electrons involved in the transition state, allowing an understanding of the type of mechanistic pathway a reaction could take.
Borges Jr team’s work uses a combination of density functional theory (DFT) methods and machine learning algorithms to calculate new Hammett constants. Whereas previous work used semi-empirical methods, Borges Jr states that the DFT methods used in this work are more accurate for calculating atomic charges. Using a variety of meta and para substituents on benzene and benzoic acid derivatives, DFT models calculated the atomic charges for the carbon atoms bonded to the groups being analysed. Processing these results with machine learning techniques resulted in the production of 219 σ values of which 92 were previously unknown.
Alongside this work, the Brazilian researchers included a set of simplified equations to obtain σ constants for new substituents that hadn’t previously been calculated. They hope that with knowledge of atomic charges obtained from other DFT calculations the simple equations can help to obtain new σ constants.
Using this machine learning approach, earlier values that had only been found experimentally were calculated computationally for the first time for three substituents (–CCl3, –NHCHO and –NHCONH2). Using DFT calculations to work out the atomic charges, these values were calculated and used as inputs for the machine learning algorithm. The resulting Hammett constants the algorithm helped to produce corresponded to literature values from experimental results for the three substituents.
Kristaps Ermanis from the University of Nottingham, an expert in computational organic chemistry, says that the work ‘can fill in values where the data hasn’t been previously found’ but that the study ‘relies on limited amounts of DFT data, which limits the number of parameters in the machine learning method, and therefore potentially also limits its accuracy’. He believes the accuracy could be easily improved in future work by acquiring more DFT data.
Matthew Grayson and his group work on computational chemistry at the University of Bath and describe the work as a ‘valuable idea that allows experimentalists to access previously unknown Hammett constants using simple and readily available atomic charge features’.
References
G Monteiro-de-Castro, JC Duarte, and I Borges Jr,J. Org. Chem., 2023, 88, 9791 (DOI: 10.1021/acs.joc.3c00410)
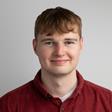
No comments yet