A machine learning algorithm tasked with designing peptides that form self-assembled structures outperformed leading experts in a head-to-head. Although the machine fell into some traps that the human experts avoided, it also discovered some highly novel peptides.
Peptide self-assembly is crucial to numerous areas of biology such as collagen formation in skin, hair and nails, and is involved in diseases such as Alzheimer’s. It also has applications outside biology. ‘Our own interest is in developing new materials for sensing like bio-electronics,’ says chemist Chris Fry of Argonne National Laboratory in the US.
Before the peptides can assemble, however, they must first aggregate in solution. This requires them to be hydrophilic enough to dissolve in water and yet hydrophobic enough to coagulate. Life uses 20 amino acids to create proteins, so the number of possible peptides of any given chain length increases 20-fold every time an extra amino acid is added. It is computationally possible to screen the properties of all 8000 tripeptides using molecular dynamics simulations, but presently impossible for the over 3 million pentapeptides.
Fry therefore teamed up with colleague Subramanian Sankaranarayanan, also at Argonne, to adapt the machine learning algorithm used in AlphaGo – which beat the world champion Lee Sedol at Go in 2015 – into an ‘AI-expert’. The crucial breakthrough of the AlphaGo algorithm was the efficiency with which it analysed all possible moves on a 19×19 board. ‘Instead of a 19×19 board game you have a problem in the sequence space,’ explains Sankaranarayanan. ‘The algorithm more or less takes the same number of evaluations regardless of how large the search space is, which means that even if your search space is several million molecules, you can still choose whatever target property you want and come up with the top-performing candidates with only a few hundred evaluations.’
The researchers started from molecular dynamics simulations of random pentapeptides, calculating the value of a metric called the ‘aggregation propensity’. They then used the machine learning algorithm to find pentapeptides with higher aggregation propensities. They performed detailed molecular dynamics calculations on the 100 best performing pentapeptides, and synthesised the nine most promising. Six aggregated in solution – a hit rate of 67%. The researchers then asked five human experts to design pentapeptides they believed would aggregate. Of the 11 that looked promising in simulations, six aggregated – a 55% success rate.
The human experts often relied on structures analogous to those known to work in other peptides and mostly stuck to four common amino acids. The AI-expert devised some highly novel, non-intuitive sequences that collectively used over 10 amino acids, as well as discovering some conventional peptides. Some of its efforts were less successful, however – it repeatedly tried to incorporate proline, which is known to break up self-assembled structures. The researchers are now working on adding additional constraints to reduce these anomalous predictions.
‘Inherently we are biased,’ says computational peptide designer Fabien Plisson of Cinvestav in Mexico City, who was not involved in the research. ‘The combination of algorithms is interesting for what they aim to do… You can see the same sort of combination of tools used for identifying anti-cancer peptides, anti-microbial peptides, anti-viral peptides – the same sort of strategies are being are being exploited in different areas of peptide research.’
References
R Batra et al, Nat. Chem., 2022, DOI: 10.1038/s41557-022-01055-3
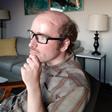
No comments yet