Two AI-driven mobile robots have been programmed to autonomously and cooperatively perform and analyse chemical reactions. A team led by Andrew Cooper, at the University of Liverpool, UK, has developed a workflow incorporating an advanced AI decision-making model that interprets data from multiple analytical tools to improve experimental design. The researchers behind the work believe it offers the potential for rapid discoveries in chemical manufacturing and drug discovery research.
In 2020, Cooper’s team introduced a mobile robotic chemist for optimising photocatalytic hydrogen production. Over eight days, the robot conducted nearly 700 experiments. However, this system was restricted to a single type of reaction and relied solely on gas chromatography for analytical feedback, limiting its ability to handle complex or diverse chemistries.
Humans and robots collaborate
Cooper’s team has now expanded the capabilities of their system to tackle three challenges in exploratory chemistry: conducting reactions, product analysis and data-driven decision making. They constructed a seamless synthesis to characterisation workflow using 1.75m tall mobile robots to operate a Chemspeed ISynth synthesis module, an ultrahigh-performance liquid chromatography-mass spectrometer (UPLC-MS) and a benchtop nuclear magnetic resonance (NMR) spectrometer. Notably, the robots are collaborative, meaning they can work alongside humans. ‘Labs are designed to be used by humans and if you hardwire things into a fixed workflow then it makes human cohabitation difficult. Here we have these instruments in the lab and students can use them while the robot isn’t,’ adds Cooper.
The synthesis module supports parallel reaction setups, thereby enabling reactions from straightforward condensations to multi-step processes. Synthesised compounds are then characterised. ‘We’re using more than one characterisation method because a lot of studies in this automated or autonomous area used NMR or MS or something else, but here we are using NMR and LC-MS together because otherwise it’s quite easy to get false negatives or false positives,’ says Cooper.
Data analysis is then used to evaluate reaction success and guide the next steps. Unlike conventional static laboratory automation, the robots operate using AI-driven logic, which allows for a human-like decision-making process that adapts to unpredictable reaction outcomes. ‘Some of the algorithmic decisions we made were relatively simple, but they were deliberately simple to be robust,’ Cooper explains. ‘We are balancing sophistication with robustness.’
As part of the workflow, a library of (thio)ureas was synthesised, which were subsequently analysed using NMR and UPLC-MS. The AI model then autonomously determined whether successful reactions should be scaled up, replicated or further modified, enabling iterative experimentation. This capability was also extended to supramolecular chemistry, where the system first used NMR to identify the characteristic chemical shifts and UPLC-MS to confirm mass-to-charge ratios, matched to theoretical predictions. ‘The workflow not only automates synthesis and analysis but also uses AI to make decisions on what steps to do next based on the analytical data it collects. It’s like a grad student that doesn’t need sleep!’ comments David Leigh, a chemist at the University of Manchester, who was not involved in the work.
Rapid decision-making
Though the system’s contextual understanding is limited, compared with human researchers, its near-instantaneous algorithmic decisions can accelerate workflows that would otherwise require lengthy manual data inspection. ‘In principle, the robots might be able to do all sorts of different chemistries, but more demanding chemistry usually requires more extensive problem solving,’ explains Leigh. In one example, while the autonomous searches yielded new chemical insights, human intervention was still needed for complex cases, such as identifying unexpected cyclisation products. ‘Whether robots and AI will be able to recognise when what they’ve observed is more important than what they were told to look for seems unlikely for the foreseeable future,’ says Leigh.
The work underscores the rapid evolution of AI and robotics in scientific discovery, complementing recent breakthroughs such as AI-driven protein structure prediction, which earned the 2024 Nobel prize in chemistry. Similar AI-driven robotic platforms are now emerging in institutions like USTC China, the Korean Institute of Science and Technology, TU Berlin and Argonne National Labs. ‘Commercial industrial robot costs have been decreasing for the last 20 years and they are still going down, whereas chemistry hardware has basically not changed at all. It’s just gone up with inflation,’ Cooper explains. ‘The reason for that is that chemistry is a very specific discipline, whereas robots are used for everything.’ The global market for AI solutions in chemical manufacturing is currently approximately £1.87 billion, but is projected to rise to nearly £30 billion by 2034.
As the team continues to refine and expand their approach, the potential for scaling these robotic systems to large industrial laboratories offers the potential for automated, intelligent chemical research. ‘Scaling this approach is feasible due to its modular design, but barriers include initial investment, personnel training and concerns about robotic hardware’s reliability in complex chemistry investigation,’ says Jie Xu, who specialises in AI-driven robotic systems at Argonne National Laboratory, US, and was not involved in the work.
References
T Dai et al, 2024, Nature, DOI: 10.1038/s41586-024-08173-7
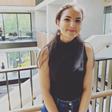
No comments yet