Machine learning models have given a never-before-seen glimpse into the intricate and mysterious mechanics of reactions driven by light–matter hybrid interactions. The techniques are a powerful new tool for scientists working in the field of polaritonic chemistry, which explores the interactions between light and matter on a quantum level.
‘Controlling a chemical reaction is quintessential in chemistry, but often we have limited control over the outcome,’ explains computational chemist Christian Schäfer from Chalmers University of Technology in Gothenburg, Sweden. ‘Polaritonic chemistry promises [to let us] steer a reaction on demand.’
In confined environments, light and matter interact to form hybrid quasiparticles called polaritons, which can be harnessed to influence chemical reactions, molecular dynamics, and different material properties.
Vibrational strong coupling, in which the internal vibrations of molecules interact with electromagnetic radiation to create an excited hybrid state, is of particular interest within polaritonic chemistry. However, developing a complete understanding of the mechanisms that drive these reactions – and providing a means of manipulating them for better outcomes – has been challenging. ‘Polaritonic chemistry is fascinating but thus far, badly understood,’ says Schäfer. ‘The precise mechanism … is still under heated debate.’ Now, Schäfer and his colleagues have used machine learning techniques to shed new light on the problem.
Current theoretical predictions have failed to provide tangible connection to experimental observations, with computational costs limiting the application of the more advanced theories needed to model these complex light–matter interactions.
‘In computational chemistry, we know perfectly well the equations we need to solve … , but their accurate solution requires an almost infinite amount of computer time,’ comments electronic structure theorist Jarvist Frost from Imperial College London, UK, who was not involved in the project. ‘Therefore, we have to make severe approximations, and are generally only able to simulate tiny systems for very short amounts of time.’
Machine learning has been reshaping chemists’ approach to computational chemistry, making it possible to predict properties and behaviours with remarkable accuracy and efficiency, enabling the exploration of systems previously deemed computationally intractable.
‘Machine learning in computational chemistry can be understood as an accelerator, allowing us to generate a thousand or a million times the amount of data, and therefore understand something genuinely new,’ says Frost. ‘You can imagine it as going from trying to explore a forest at night with a tiny pencil torch and just seeing the moss underfoot, to turning on a big flashlight and being able to see the trees and the wood on the hill beyond.’
A smoking gun for polaritonic chemistry
Schäfer and his colleagues tested their approach on a well-understood reaction. ‘The deprotection reaction of 1-phenyl-2-trimethylsilylacetylene has been among the first well-studied experimental works in polaritonic chemistry,’ says Schäfer. ‘Understanding the involved mechanism would serve as a smoking gun for polaritonic chemistry and would foster its development.’
To train the model, Schäfer’s team generated numerous configurations of the 1-phenyl-2-trimethylsilylacetylene molecule that would exist during the reaction and provided estimates of its energy, force, and optical characteristics that were calculated using conventional density functional theory.
‘The machine learning model is then educated with this information and will slowly learn how to connect a given configuration of atoms with, [for example], an energy,’ explains Schäfer. ‘Teaching takes time and energy … but a well-educated model can pay back the initial cost many times.’
For the first time, the model provided a direct correlation between theoretical prediction and experimentally measured changes in chemical kinetics, revealing the influence of single-molecule strong coupling on the silicon–carbon bond during the reaction. It also demonstrated a frequency-dependent rate constant – a crucial aspect of polaritonic chemistry – that aligns with experimental observations of changes in enthalpy and entropy. Notably, both inhibiting and catalysing effects were observed for the same reaction, contradicting previous computational calculations.
‘This is very exciting, if true,’ says Frost. ‘Obviously, the work needs to be validated to ensure that their machine learning method is accurate. A major issue with these machine learning methods is in validating the output. It is very difficult to quantify the errors.’
Though still early days, Schäfer believes that the work offers a clear path for future development. ‘We hope it will trigger a change in paradigm on … circulating ideas for the fundamental mechanism behind polaritonic chemistry.’
References
C Schäfer et al, JACS, 2024, DOI: 10.1021/jacs.3c12829
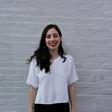
No comments yet