A data-driven map has been formulated that models the link between the chemical structure of an odourant with its smell. The researchers hope the tool will pave the way towards eventually being able to store odours digitally, as is possible with images and sound.
Unlike sight and hearing, there is no universally accepted method for quantifying and categorising how we experience smells. Although several systems of classification have been proposed, none have gained widespread acceptance.
In an attempt to rectify this, the researchers constructed a specific type of graph neural network, called a message passing neural network, to generate a principal odour map (POM) linking chemical structures to their smell.
On the map, each molecule was represented as a graph, with each atom described by a number of criteria such as its valence, hybridisation and atomic number, and each bond described by its number of neighbours, aromaticity and whether or not it’s in a ring.
To test whether the model extended to novel odorants, the researchers compiled a list of potential odorants whose empirical properties are currently unknown to science or industry – most of which have never been synthesised before – and plotted them into the POM to get an idea of how they might smell. They then tested the efficacy of the POM on these odorants against humans who had been specifically trained beforehand.
‘We wanted to do a prospective validation,’ explains Joel Mainland, an olfactory neuroscientist at Monell Chemical Senses Center in Philadelphia, US and one of the authors of the study. ‘We picked 400 molecules from a drug screening library, most of which no one had ever smelled before… Then we had a panel of human subjects smell them and rate them, and then we had the computer model predict what they would smell like.’
The researchers found that the model was as reliable as the median human panellist in describing odour quality and outperformed chemoinformatic models on several other odour prediction tasks. The model performed best for labels such as ‘garlic’ and ‘fishy’ that have clear structural determinants (sulfur-containing moieties and amines, respectively) and worst for labels like ‘musk’ which include at least five distinct structural classes (macrocyclic, polycyclic, nitro, steroid-type and straight chain).
The researchers’ ultimate goal is to be able to digitise odours, in the same way that images and sounds can be digitised and then stored in an archive. ‘There are lots of applications for digitising smells,’ says Mainland. ‘[For example] most of the perfume industry has stopped making novel molecules, because … you need a lot of safety testing to take a new molecule and put it in production. And so what [digitising] lets you do is find many different molecules that have the percept you want and figure out which ones are best in terms of cost and performance and safety.’
The team are now also looking at mixtures of odours, as well as single molecules, to enable predictions to be made as to what odours will result from mixing specific components.
‘You could, for example, create primary odours the same way we had primary colours and understand how to make mixtures,’ Mainland adds.
Kobi Snitz, a brain imaging statistician based at the Weizmann Institute of Science in Israel, describes the study as an ‘important contribution to a fundamental problem in olfaction’. ‘The authors applied an advanced neural network model to this complex problem and achieved a milestone result. A data driven representation of the space of odorants which is informative across data sets and across modelling tasks,’ he says.
‘This work represents an important step in the effort to parametrise the space of odours in a similar way to how audition and vision are parametrised.’
References
B Lee et al, Science, 2023, 381, 6661 (DOI: 10.1126/science.ade4401)
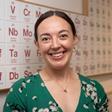
1 Reader's comment