A novel neural network can help chemists calculate adsorption energies effectively and efficiently – up to one million times faster than state-of-the-art methodologies. Once again, artificial intelligence has proven to be a potent tool to accelerate discovery, particularly in the field of heterogeneous catalysis. The improvements in the design and development of catalysts could create cleaner chemical processes, and enable the exploration of innovative raw materials such as biomass and plastics.
‘[Our neural network is] a catalyst for heterogeneous catalysis,’ says Núria López from ICIQ in Tarragona, Spain, who co-led the study. Traditionally, computational chemists calculate adsorption energies with density functional theory (DFT), which have several limitations. ‘DFT is really time consuming, plus it doesn’t really work for very large molecules,’ she adds. ‘The new neural network algorithms predict the adsorption energy of big molecules on metal surfaces orders of magnitude faster,’ explains López. In heterogeneous catalysis, activity is actually associated with adsorption energy. Eventually, estimating the energy of adsorption accurately could lead to more efficient experiments in the lab.
One of the tricks was representing molecules as mathematical ‘graphs’ – structures with several nodes interconnected by links. Within this simple system, nodes symbolise the atoms of different elements, and links illustrate chemical bonds. ‘It’s a natural representation of molecules, much like using balls-and-sticks models,’ says López. The concept had already been adopted in molecular simulations, but remained relatively unexplored in heterogeneous catalysis because ‘it was hard to simplify the structure of the surfaces’. Researchers solved this challenge by creating graphs for the catalytic surfaces – selecting the smaller number of atoms in contact with the molecules.
The team trained the algorithm, which was dubbed GAME-Net, on small molecules, featuring fundamental functional groups like amines, amides, esters and aromatics – among others. For the simulation of surfaces, the dataset included 14 metals with different facet frameworks. ‘We had calculated the adsorption energies of small molecules with DFT to nourish the neural network,’ adds López. ‘The algorithm swiftly learns the basic concepts of chemistry, and becomes competent to calculate the adsorption energy of big molecules with minimal errors,’ she explains.
The new neural network is ‘an exciting tool in the world of heterogeneous catalysis’, says Nong Artrith, an expert in machine learning and computational chemistry at the University of Utrecht in the Netherlands. ‘It overcomes the long-standing challenge of modelling the interaction of large molecules with catalysts’ surfaces … and predicts adsorption energies with lightning-fast speeds,’ she adds. The new neural network could drive the discovery of catalysts for biomass conversion, leading to the sustainable production of chemicals from renewable resources. ‘Compared to DFT, the speed and accuracy of the model are very impressive,’ adds Artrith. ‘This innovation paves the way … towards a cleaner and greener future.’
Although ‘adsorption energies often correlate with catalytic activity, [further steps] could test the trends in experimental laboratories to compare with the predictions of the neural network’, comments Artrith. López explains that accurate experimental measurements of adsorption energies with traditional thermochemical techniques takes a tremendous amount of time, and few experimental databases are available for comparison purposes. However, the team hopes to promote partnerships to explore experimental endeavours in the near future.
Furthermore, researchers will soon open the tool to the chemical community, creating a user-friendly website that works with simple inputs such as drawn structures, Smiles strings, PubChem numbers and molecule names. Then, the site speedily simulates the adsorption energy to the selected metal surface. ‘Open-access and collaboration have been at the heart of this project, a joint effort of experts in Canada, Switzerland and Spain,’ explains López. ‘Usually, with DFT, a single simulation of adsorption energy for a large molecule could take days in a supercomputer,’ she adds. ‘Thanks to our GAME-Net neural network, now all you need is laptop.’
References
S Pablo-García et al, Nat. Comput. Sci., 2023, DOI: 10.1038/s43588-023-00437-y
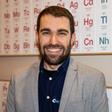
No comments yet