Exposing new dimensions in the relationships between elements
How are you enjoying the International Year of the Periodic Tables so far? Yes, tables – we should probably have been using the plural all along. Since Dmitri Mendeleev (and others) first sketched out the periodic relationships between the elements in the 1860s, it has been estimated that around a thousand different tables have appeared in print – and that’s before considering all those on the internet. Even the T-shirts handed out at the opening ceremony in January (I grabbed one, naturally) offered a new version, courtesy of the European Chemical Society, with the elements colour-coded and given different-sized boxes according to their abundance and availability.
Mostly these tables embody careful deliberation about what to put where, which information to prioritise, which message to convey. But two recent papers have shown that it is now possible to rediscover the table empirically, from the way it is implicitly embedded within the milieu of chemistry.
Periodic_table v2.0
Both methods use machine learning (ML): the standard form of most artificial-intelligence algorithms at present, in which relationships and correlations between variables are deduced by combing through data. These schemes can often identify connections invisible to humans, because we can’t generally process that much data and because the correlations may exist in high-dimensional spaces that we cannot visualise. Michele Ceriotti at the École Polytechnique Fédérale de Lausanne and his coworkers have applied ML to crystal structures drawn from a dataset1 of around 11,000 quaternary compounds of the type ABC2D6, calculated for a wide range of compositions (incorporating 39 main-group elements) by density functional theory. The structures are represented as a vector of features that describe both the geometric relations between different atoms and their chemical identity.
The exercise suggests that the periodic table has more dimensions than the page – based solely on the chemistry of the elements
They then simplified these high-dimensional descriptions by mapping the elements onto a compressed, lower-dimensional space in which each element is characterised by values of a handful of abstract quantities. It’s a little like the old classical idea that the substances we see, such as iron or copper, are made up of the more fundamental ‘elements’ earth, air, fire and water, combined in different proportions. Ceriotti’s team has used other datasets too, producing other representations of the elemental relationships.
In these representations, certain elements are found to cluster together in the low-dimensional space. An obvious question is whether the proximity of elements in these projections matches the relationships found in the periodic table. Ceriotti and colleagues answer that question with an easily eyeballed, graduated colour-coding of elements in the conventional format of the table such that similarities are reflected in their hue.2
The projections typically colour the columns much as we’d intuitively expect: for example, the noble gases appear almost identical to one another and distinct from all the others, the halogens tend to form a monochrome column, and the alkali metals too have a unity that is close in colour to that of the alkaline earth metals. But in some tables these boundaries are more revealing. Fluorine can look rather different from the other halogens; indeed, the whole of the first p block row may display a character distinct from those below. Hydrogen is consistently anomalous at the top of group 1.
The reverse process – colouring the low-dimensional plots themselves according to the conventional element groups – has a similar effect. For the most part, elements of the same group are all found together, but the exceptions show where the algorithm has divined some difference in character for an element in the context of a particular dataset. For example, a two-dimensional plot obtained using data on perovskite stability places the group 1 elements close together, but hydrogen’s ability to act as a hydride (of which the machine has no understanding) makes it slippery and puts it in the neighbourhood of halogens and chalcogens. If the plot is extended to a third dimension, the algorithm compromises somewhat, moving hydrogen and the alkali metals ‘up’ out of the plane to approach each other.
All of this feels right, wouldn’t you say? The exercise both reaffirms the traditional groupings of the periodic table and reminds us of the subtle distinctions that cut across it, the individuality of certain elements. It suggests that the table has more dimensions than the page – based solely on the actual chemistry of the elements.
Literature review
The ML approach taken by Vahe Tshitoyan at Lawrence Berkeley National Laboratory (LBNL) in California and colleagues is even more bold.3 The LBL team has long spearheaded the Materials Project, an effort to collect a massive database of the properties and structures of known materials that might be mined using supercomputers to spot structure–function relationships and predict potentially useful new materials. One of the challenges for exercises like this – other initiatives and companies elsewhere are seeking to exploit similar methods for materials discovery – is that there is no standardised and easily accessed format in which such data are encoded in the literature.
The researchers have turned this problem into an opportunity. They have used ML to look for correlations between the actual words used in published papers within their materials science dataset. Algorithms already exist for analysing texts this way, and have been used previously to look for trends in literature and historical documents, without needing guidance from human supervision. Tshitoyan and colleagues applied these methods to 3.3 million abstracts of papers in materials science published between 1922 and 2018, spanning a vocabulary of half a million ‘words’ – some of which are in fact chemical formulae. The analysis revealed expected correlations such as, for example, that between ‘NiFe’ and ‘ferromagnetic’, or ‘Bi2Te3’ and ‘thermoelectric’.
That much seems like a fancy way of rederiving things we have discovered already. But sometimes a material might be studied and characterised without the researchers realising what useful applications the properties could suggest. Tshitoyan and colleagues show that many top-performing thermoelectric materials, for example, could have been predicted this way several years in advance of the actual discovery of that potential.
And here too, the chemical elements are grouped in ways that reflect their periodic relationships. For example, in the vast space of word associations the halogens are neatly grouped together, although when that grouping arises from the term ‘diatomic nonmetals’ they are then united with nitrogen and oxygen too. (Astatine is, quite properly, the outlier.) The transition metals are all gathered together, and are distinguished from, say, the post-transition metals like aluminium and tin.
This much is perhaps not so surprising: it simply shows that our traditional classifications of the elements are firmly embedded lexically in the chemical literature. But they are so presumably because we find that convenient and useful. To put it another way: we might argue all we like that the ‘right’ place for hydrogen can only be above lithium, or that lanthanum belongs in the d block and not the f block – but normative chemical practice as revealed in the literature seems to tell us that we should not pay too much heed to such suggestions.
This returns us to a fundamental question about those thousand-plus periodic tables. Are we obliged to make a single, optimal choice based on objective facts, or can we be pragmatic pluralists? In his new edition of The Periodic Table: Its Story and Its Significance, updated for the IYPT, Eric Scerri calls the first group realists (in the philosophical sense), the second instrumentalists or ‘anti-realists’. To realists, our groupings of elements reflect the way nature itself is ‘carved at the joints’. Our chemical intuition surely suggests that some divisions – the noble gases, say – are like that (although such joints seem likely to be blurred for the superheavy elements). But is the entire periodic table truly carved at the joints? To judge from these two studies, both chemical properties and actual disciplinary usage recommend various ways of making the cuts: some joints are clearer than others.
References
1 F A Faber et al., Phys. Rev. Lett., 2016, 117, 135502 (DOI: 10.1103/PhysRevLett.117.135502)
2 M J Willatt, F Musil and M Ceriotti, Phys. Chem. Chem. Phys., 2018, 20, 29661 (DOI: 10.1039/c8cp05921g)
3 J Schmidt et al., Chem Mater., 2017, 29, 5090 (DOI: 10.1021/acs/chemmater.7b00156)
4 V Tshitoyan et al., Nature, 2019, DOI: 10.1038/s41586-019-1335-8
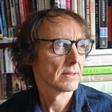
No comments yet