A new AI system, dubbed InstaNovo, could revolutionise protein sequencing just as AlphaFold transformed protein structure prediction, its developers claim.
While DNA sequencing is routine, determining protein sequences remains one of biology’s toughest challenges, says corresponding author Timothy Jenkins of the Technical University of Denmark. InstaNovo aims to change that by directly reading protein sequences from raw experimental data, unlocking vast areas of previously inaccessible biology.
In proteomics – the study of proteins in biological systems – de novo peptide sequencing is used to figure out a protein’s base amino acid sequence using tandem mass spectrometry. This technique fragments peptide ions and analyses their mass-to-charge ratios at multiple stages, allowing researchers to infer the original sequence.
‘There are many techniques for studying proteins, but none match the throughput and comprehensiveness of mass spectrometry,’ says Kostas Kalogeropoulos, also at the Technical University of Denmark. ‘We analyse proteins – or their smaller fragments, peptides – by measuring their mass.’
Unlike database-dependent approaches, which compare unknown peptides to known sequences, de novo sequencing reconstructs them from scratch, requiring no prior information. ‘De novo sequencing has long been underappreciated, yet it holds immense potential for many biological applications,’ says Jenkins. However, issues with accuracy and high computational costs have hindered its widespread adoption.
‘Traditionally, de novo peptide sequencing relies on algorithms that functioned similarly to manually reconstructing a sequence,’ explains Kalogeropoulos. ‘These methods require all necessary information to be present, otherwise they would fail.’
‘InstaNovo and similar tools circumvent this major limitation by direct “de novo” interpreting peptide sequences from peptide fragmentation spectra,’ comments Francis Impens at the VIB research institute and the University of Ghent, who was not involved in the study. ‘That means we can now identify proteins from genomically unsequenced species or from very complex samples, [such as] microbiome samples, for which the species composition is unknown.’
InstaNovo is a transformer-based AI, a neural network originally used in language processing that learns context and meaning by tracking relationships in sequential data – like the correct sequence of words in a sentence.
When applied to de novo peptide sequencing, InstaNovo analyses peaks or signals from mass spectrometry data and processes them through multiple steps using transformer decoder layers, which are like smart filters that piece together the most likely sequence of amino acids from the fragmented data.
To choose the most accurate sequence, InstaNovo uses a ‘knapsack beam search’, a strategy that efficiently tests different possible sequences, keeps the best ones and then refines them. This works similarly to how a human would double-check and fine-tune their guesses when manually sequencing proteins.
‘InstaNovo … directly predicts the sequence from the spectrum, eliminating the need for database lookups,’ says first author Kevin Eloff. ‘This is possible because our models have learned the underlying patterns of the sequences we are measuring and can translate a spectrum directly into the corresponding peptide sequences.’
As a proof of concept, InstaNovo was used to analyse peptides in fluid from patients’ wounds, identifying at least three pathogens, which were confirmed by standard techniques. ‘While the presence of pathogens in these wounds was not unexpected, we were surprised by how easily we could detect them,’ says Kalogeropoulos. ‘This finding could have significant implications for how we diagnose and treat chronic wound patients.’
The team is now exploring InstaNovo’s potential to map all the proteins in a patient’s cell. It could also identify mutated cancer proteins and proteins with unknown roles.
Impens finds it exciting that InstaNovo can extend database search results beyond known sequences, surpassing previous de novo sequencing tools. However, it still needs to be evaluated and trained on larger datasets.
‘The model still needs to be fine-tuned for post-translational modifications [which affect protein function and are critical for biological activity] and data from different types of mass spectrometers,’ he adds.
As with any new technology, he expects challenges in integration and real-world application. Jenkins and Kalogeropoulos agree but believe that interdisciplinary collaborations will demonstrate the model’s benefits.
‘We cannot say that de novo peptide sequencing is fully solved yet,’ says Eloff, ‘but we hope to train on a lot more data and make improvements wherever we can … making state-of-the-art models available to anyone’.
References
K Eloff et al, Nat. Mach. Intell., 2025, DOI: 10.1038/s42256-025-01019-5
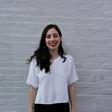
No comments yet