A new algorithm can predict the bitterness of a compound using only mass spec data, without knowing the chemical’s structure. The team from Hebrew University in Jerusalem says this new tool, dubbed BitterMasS, could streamline compound screening processes in food science, drug development, metabolomics, and other areas. The scientists also say it marks a significant improvement over traditional methods that rely on structural data.
The team trained a machine learning algorithm on a dataset of more than 5400 mass spectra of bitter and non-bitter compounds. The tool was then able to identify bitter compounds without structural information, exhibiting 67% precision in one test.
This appears to be the first attempt to predict the taste of a compound from mass spec data, the team says. The researchers suggest that BitterMasS could, for example, monitor bitterness changes over time and offer important insights into food quality, as well as safety, as bitterness can be linked to toxicity.
‘BitterMasS represents a critical shift in taste prediction,’ stated the study’s lead researcher Masha Niv. ‘By leveraging mass spectrometry data, we can now predict bitterness directly and efficiently, opening doors to new discoveries in health-promoting compounds and enhanced food processing techniques.’
Where the chemical structures are unknown, the team concludes that it has demonstrated that predicting bitterness directly from mass spec data is more efficient than a traditional strategy that needs the intermediate step of structure determination before predicting bitterness.
References
E Ziaikin et al, J. Agric. Food Chem., 2024, DOI: 10.1021/acs.jafc.3c09767
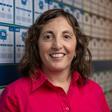
No comments yet