Protein structure prediction is a hard problem, but even harder ones remain
DeepMind’s AlphaFold team has been having quite a run of success in predicting protein structures. This has long been considered one of the truly difficult problems in computational biology, and AlphaFold has made extraordinary progress over the last year or two. This has culminated in the recent release of predicted structures for the great majority of the human proteome, which is the sort of thing that, ten years ago, would have sounded like the opening of a science fiction story.
I have no desire to take anything away from this success. It really is impressive. But some of the headlines have betrayed real misunderstandings about what’s been accomplished. First off, as I wrote here earlier this year, we have not made sudden huge leaps in understanding why proteins fold like they do. The AlphaFold people have made great progress in recognising different known protein folding motifs and assembling them into structures that are very often correct. Forming these coils, loops, and sheets is what proteins generally do, but ‘why?’ doesn’t enter into it. If we’d waited for an answer at that level, we’d be waiting for many more years.
It is very, very rare for knowledge of a protein’s structure to be any sort of rate-limiting step in a drug discovery project
Instead, we have thousands and thousands of new protein structure predictions, well before we might have expected them. And they really do seem to be mostly correct, albeit with some exceptions. Some of the structures are more solidly determined than others, but then some proteins are intrinsically more determined than others. AlphaFold’s algorithms are at a loss when confronted by disordered protein regions, as well they might be: when your entire computational technique is built on finding analogies to known structures, what can you do when there’s no structure to compare to and never will be? Some disordered proteins snap into orderly arrangement in the presence of their various protein partners, but others never show ordered structures under any conditions. What’s more, that property seems to be essential to their function! Some protein behaviour is simply beyond structure, and thus beyond AlphaFold’s ability to help.
It also needs to be emphasised that (as carefully worded above) what we have are predictions and not real protein structures. They’re good predictions and useful ones, but obtaining actual data (from x-ray, NMR, or cryo-electron microscopy) is the only way to be sure how correct they are. And because of conformational flexibility, even those aren’t always the last word. That’s why press coverage about how this new prediction database will revolutionise drug discovery are overblown. Protein structures shift and slide in the presence of small-molecule ligands, sometimes subtly and sometimes dramatically, but AlphaFold is not (yet) equipped to predict these changes. We may well be able to find algorithmic solutions to those questions eventually, but as yet there simply aren’t enough small-molecule ligand-bound protein structures to build from. We’ll need a lot of them. There are twenty or so different protein side chains to account for, but the number of small molecule structures is so huge as to be practically infinite by comparison.
The protein’s structure might help generate ideas about what compounds to make next, but then again, it might not
And there’s another point, which is going to sound heretical (although it’s true). It is very, very rare for knowledge of a protein’s structure to be any sort of rate-limiting step in a drug discovery project! That’s because you’re almost always running the project based on assays using the pure protein or with living cells. Those numbers are supposed to answer the key questions: are these compounds doing what we want, and are they getting better as we make new ones? The protein’s structure might help generate ideas about what compounds to make next, but then again, it might not. In the end the real numbers from the real biological system are what matter. As a project goes on, those numbers include assays covering pharmacokinetics, metabolism, and toxicology, and none of those can really be dealt with from the level of protein structure, either.
After those rapids comes the final waterfall. In the end, drugs fail in the clinic because we have picked the wrong targets or because they do other things that we never anticipated. Protein structure by itself does nothing to mitigate either of those risks, but those are why we have an 85% clinical failure rate in this business. Protein structure is (was?) indeed a very hard problem. But guess what? These are even harder.
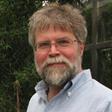
9 readers' comments